By Tom Paquin
I’ve been pleased and proud of the content that The Future of Field Service has delivered over the course of the last few months to help support businesses as they navigate the COVID-19 crisis and beyond. Through shaky economic times, fear, and uncertainty, I hope that we have been able to provide some guidance and some optimism for how we, as a service community, can emerge from this crisis with a new focus on transformative service delivery.
Having said all that, there are obviously two sides to the crisis. One is tackling these challenges head-on in writing, advisement, and adjustments to the way we do business and live our daily lives. The other is filling the wealth of time that we have not sitting in traffic, not going out to eat, and not puttering around Target for a few hours on a Sunday afternoon.
For me, that extra time has been filled in three ways:
- Working earlier/later/weirder hours than usual
- Remodeling a bathroom
- Playing video games
The third of these things (I play quite a bit of Sid Meier’s Civilization 6, in case you’re curious about just how big of a nerd I am) reminded me of an article that I wrote a few years ago while at Aberdeen about the relationship of AI in video games and the world of service. In the opening of that article I said:
Back in 2002, developer Monolith Productions was showing off a technical demo of the AI systems in its upcoming game, No One Lives Forever: A Spy in H.A.R.M.’s Way. The Artificial Intelligence in this game was a pretty substantial leap forward compared to its contemporaries. Rather than simply stand around until the player character walked by, in this game, non-player characters would behave, seemingly, like real people. They’d step outside for a cigarette, engage in idle chatter, go to a nearby soda machine for a drink, and even use the bathroom (characters inside would use toilets, characters outside would find the nearest bush).
Monolith’s head of AI, Jeff Orkin, demonstrated how characters would make dynamic adjustments to environmental changes. He identified a character heading towards the bathroom, and before they arrived, Orkin dropped a grenade in the toilet and blew it up. The guard stepped into the bathroom, saw there was no toilet, and walked back out into the hallway. Then, he dutifully walked up to a nearby potted plant and relieved himself there.
This somewhat embarrassing example illustrated the concept of emergent gameplay, taking three elements—AI goals, the external environment, and player input—and using them to determine a set of actions. The behavior of the character in that instance was built off of a system known as goal-oriented action planning, or GOAP. GOAP replaced more linear automation systems in the early 2000s in video games.
The amazing thing about goal-oriented action planning is that even though it’s a concept borne out of the controlled world of video games, it has a direct connection to service system as well.
The basic concept of GOAP is taking traditional automation inputs and making them more efficient. Traditional process automation strings together a set of goals in a sequence. For example, imagine that your service technician is an AI (This is just an example…I’m not advocating for the use of robo-technicians). Using traditional automation, their sequence of daily actions looks like:
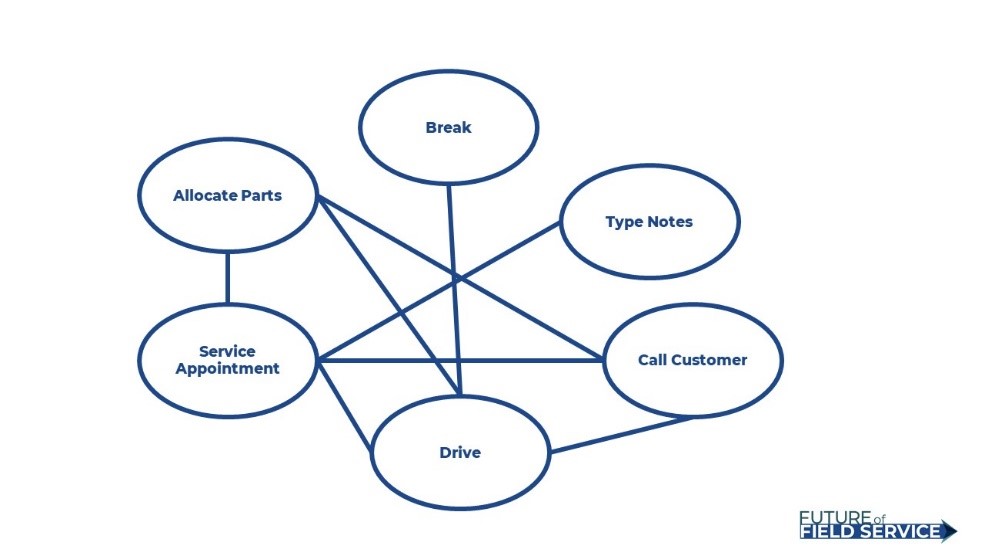
So each behavior (the circles) need to emanate directly along a specific path in order to be completed. There is no direct line between “Service Appointment” and “Break”. You need to pass through “Drive” in order to get there, meaning that a technician would need to drive to their break. “Type Notes” only connects to “Service Appointment”, so it needs that behavior to have been completed in order to be initiated.
See the issue with this?
The biggest problem that programmers run into with traditional automation when it comes to video games is that introducing a new behavior to an AI (Remote assistance, for instance) creates dozens of broken logic chains unless you go back and remap all the paths above, and even so, the pathways are so finnicky that they often break themselves. What happens, for instance, if your technician has two appointments at the same site? What if your technician needs to reallocate parts? What if they decide to eat a sandwich in their truck? What if they call a customer who has to reschedule? What if a technician doesn’t have time to type notes before their next appointment? The sequence is too rigid.
That is why, in contrast, goal-oriented action planning looks like this:
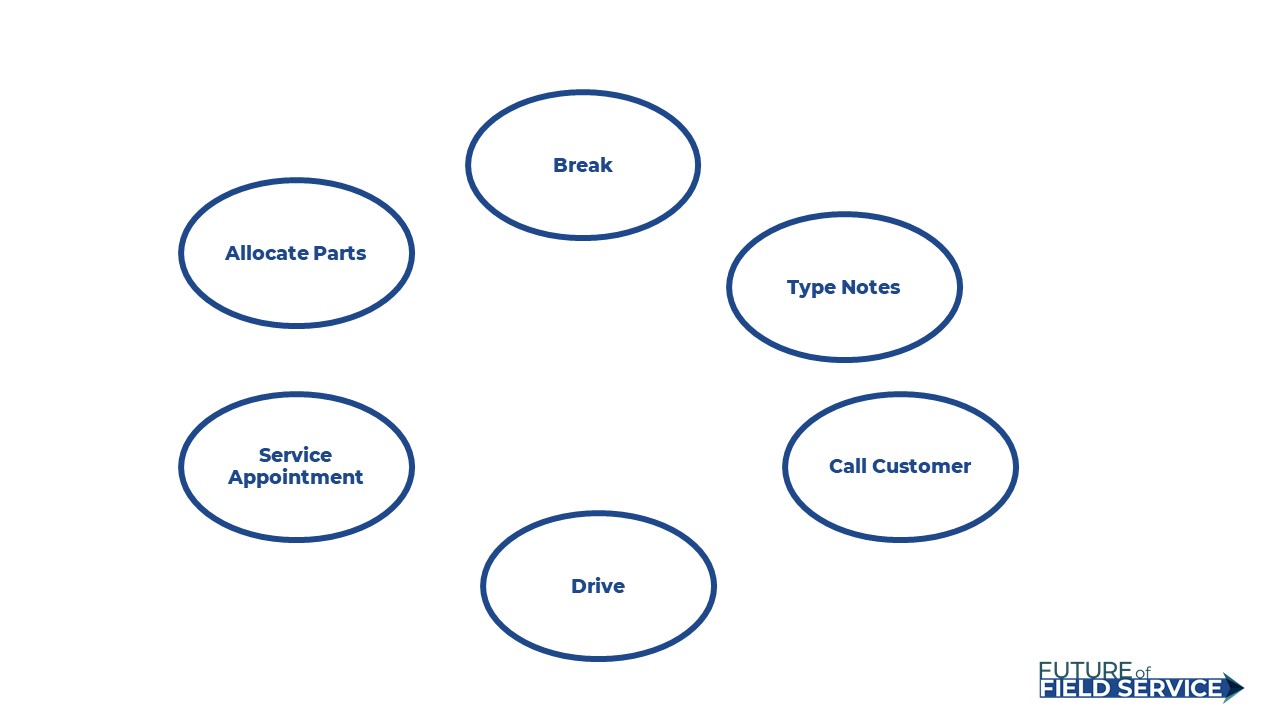
Same behaviors, no path. Instead, the AI evaluates the current conditions, and makes an evaluation to initiate any of its available behaviors based on that condition. This naturally leads to improved efficiency, often beyond what programmers had intended, which explains how a security guard ends up relieving themselves in a plant in the example I wrote about above.
I imagine you might be a bit skeptical, especially since the theoretical utilization of goal-oriented logic is what led HAL to murder everyone in 2001: A Space Odyssey. This is where simulation comes in. These AI systems, by design, run a scenario thousands of times, each time “evolving” their approach to produce a more efficient outcomes.
How do you make sure those “evolutions” don’t turn murderous? Or make sure your technicians have time to eat? You set rules. Let’s take this example away from an individual tech and talk about an actual, practical use of AI: Planning and Scheduling. To optimize scheduling (as I spoke about just last week), you give locations of specific objectives, define the criteria that needs to be met to accomplish those objectives (parts needed, safety precautions, required breaks, hours of operation, travel time, available staff, etc) and you allow the systems to find the most efficient way to complete all of their goals within the context of the rules. Further, you can rank goals, and you can update in real-time.
This is how the best PSO systems function, with AI under the hood doing the work for you. Most can do that for your day. The best are able to provide multi-time horizon planning that takes into account an extremely wide variety of factors and can map well in advance.
Under most normal circumstances, developing this system will not be the job of the deliverer of service, but it’s nevertheless a useful concept to understand, especially when evaluating a PSO solution, not to mention thinking about asset management, or any other number of systems that will take advantage of AI. By understanding its capabilities, you can plan your criteria, inputs, and goals appropriately to take full advantage of what this amazing technology has to offer. And maybe it’ll make you waste a few less coins the next time you boot up Pac-Man.